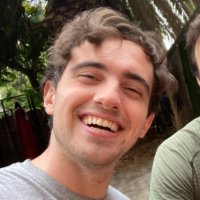
Chris Painter
@chrispainteryup
Evals accelerationist, working hard on responsible scaling policies, policy director @METR_Evals
ID: 930159122535866369
13-11-2017 19:43:11
878 Tweet
1,1K Takipçi
1,1K Takip Edilen

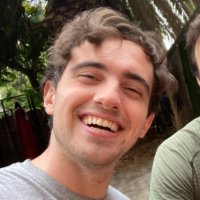
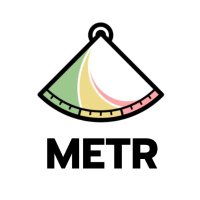
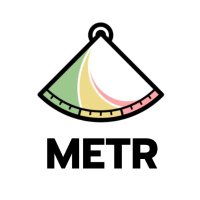
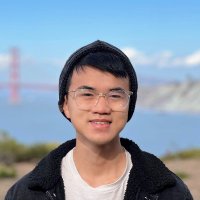
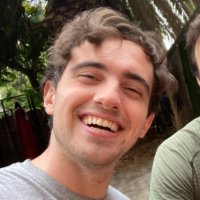
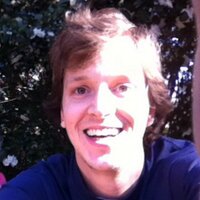
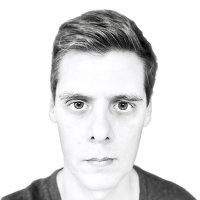
Can’t wait for the amount of resources Casey Handmer, PhD will put into antimatter production and storage after Terraform exits caseyhandmer.wordpress.com/2024/08/18/ant…
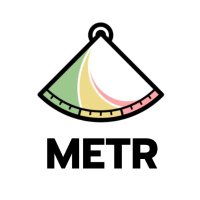
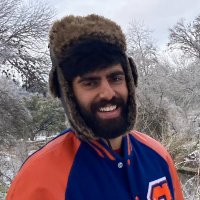

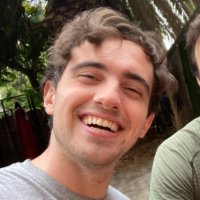
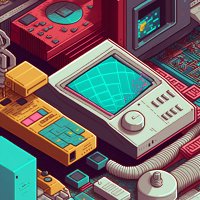
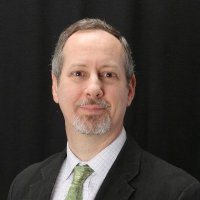
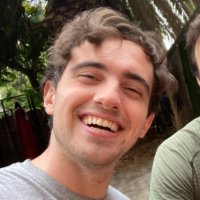
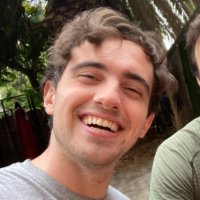
A few months ago I hosted a Q&A with Geoffrey Hinton in Berkeley: youtube.com/watch?v=PTF5Up…
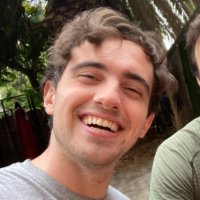
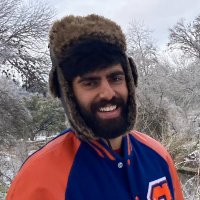
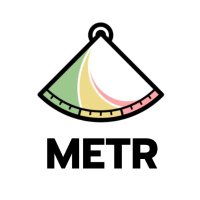