
Zirui "Colin" Wang
@zwcolin
cs @princeton_nlp @princetonPLI | prev @HDSIUCSD @CogSciUCSD, @CarnegieMellon. synergize model understanding & generation; multimodality; He/Him.
ID: 2986434572
http://ziruiw.net 17-01-2015 04:18:40
76 Tweet
446 Followers
375 Following
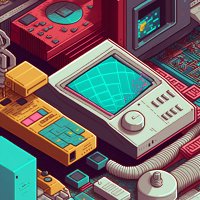
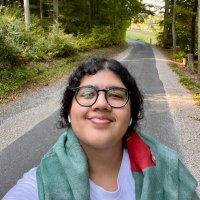



