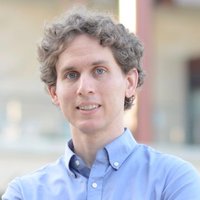
Jacob Steinhardt
@jacobsteinhardt
Assistant Professor of Statistics, UC Berkeley
ID: 438570403
16-12-2011 19:04:34
329 Tweet
7,7K Takipçi
69 Takip Edilen
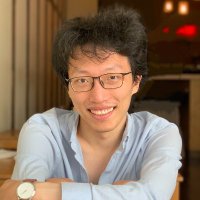
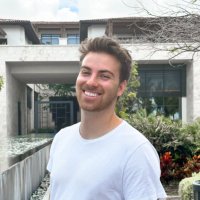
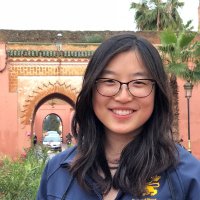
Protein language models (pLMs) can give protein sequences likelihood scores, which are commonly used as a proxy for fitness in protein engineering. But what do likelihoods encode? In a new paper (w/ Jacob Steinhardt) we find that pLM likelihoods have a strong species bias! 1/
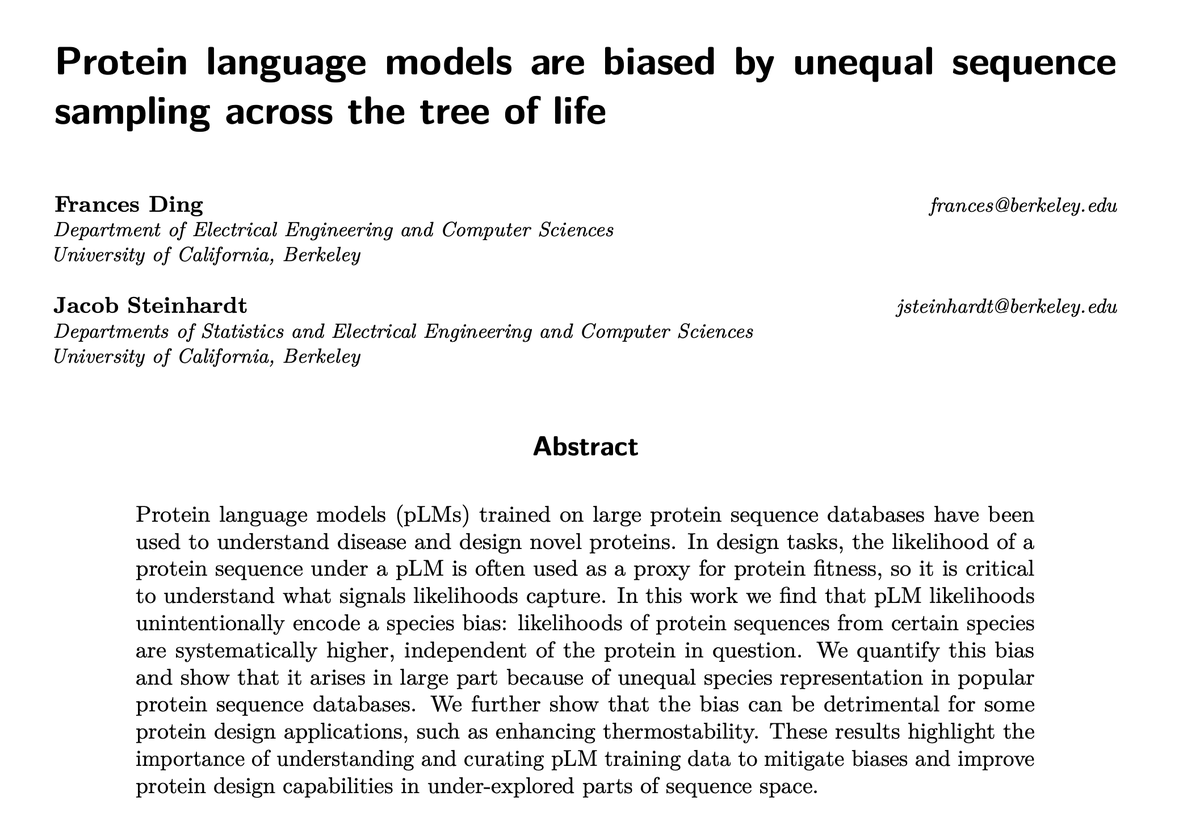
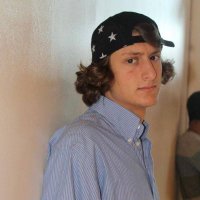
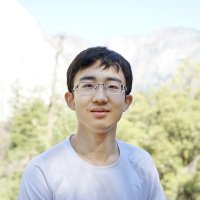
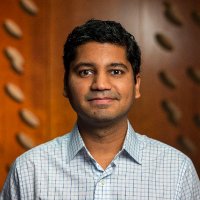
In a new preprint with Jarek Blasiok, Rares Buhai, David Steurer, we show a surprisingly simple greedy algorithm that can list decode planted cliques in the semirandom model at k~sqrt n log^2 n --essentially optimal up to log^2 n. This ~resolves Jacob Steinhardt's open question.
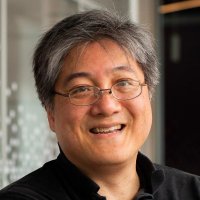
I am delighted to officially announce the National Deep Inference Fabric project, #NDIF. ndif.us NDIF is an U.S. National Science Foundation-supported computational infrastructure project to help YOU advance the science of large-scale AI.
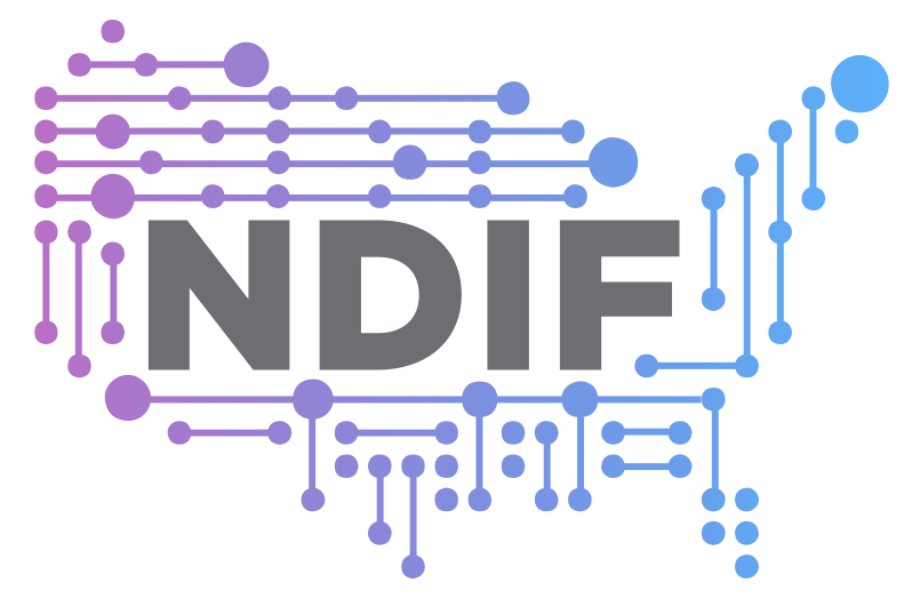
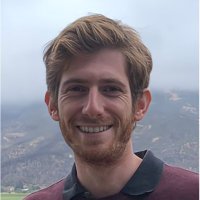
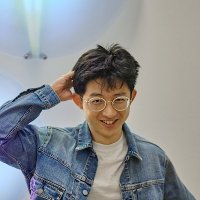
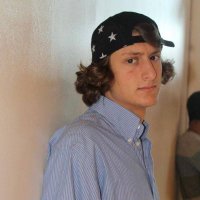

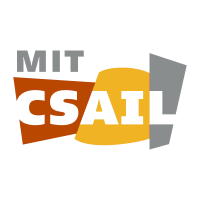