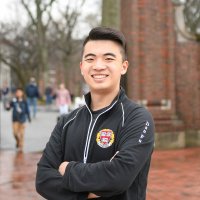
Dan Fu
@realdanfu
Incoming assistant professor at UCSD CSE in MLSys. Currently recruiting students! Also academic partner @togethercompute.
ID: 1173687463790829568
http://danfu.org 16-09-2019 19:58:03
611 Tweet
5,5K Followers
183 Following
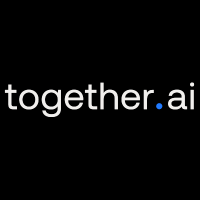
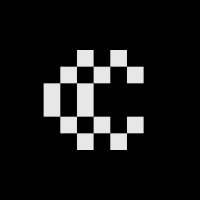

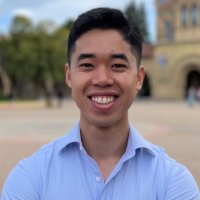
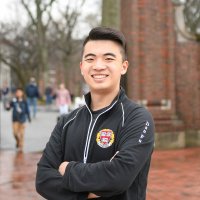
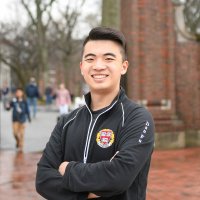
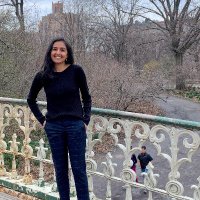
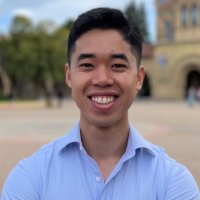
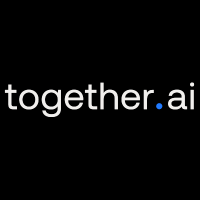
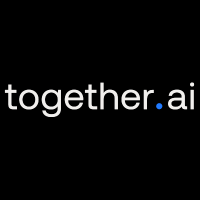
Our Llama 3.1 405B endpoint provides the best decoding performance by a huge margin, via Artificial Analysis Try it here: api.together.xyz/playground/cha…
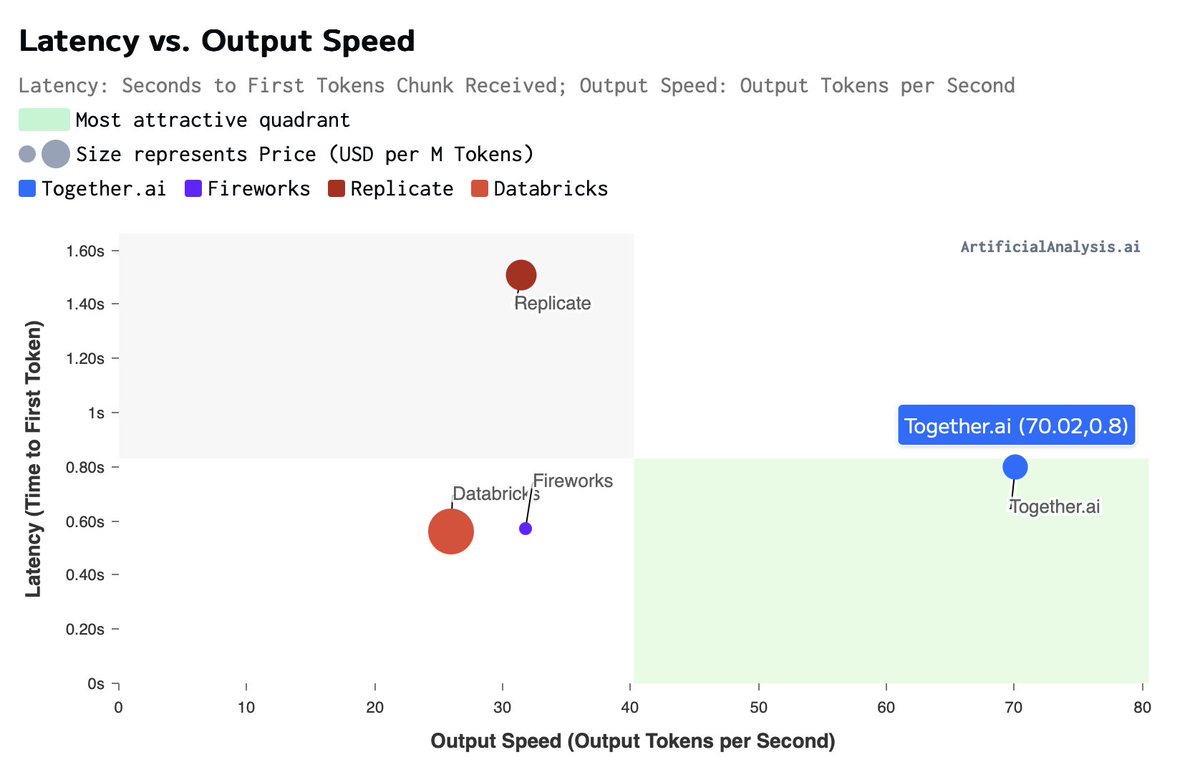
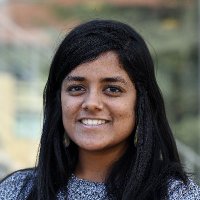
