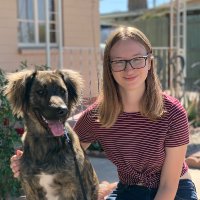
Amanda Bertsch
@abertsch72
PhD student @LTIatCMU / @SCSatCMU, researching text generation + summarization | she/her | also @ abertsch on bsky or https://t.co/L4HBUh0R9f or by email (https://t.co/bsHqwIMFPL)
ID:2780950260
https://www.cs.cmu.edu/~abertsch/ 30-08-2014 19:05:43
268 Tweets
1,3K Followers
726 Following

Happy Halloween! Be careful out there. Look what I found inside some trick-or-treat candy.
(Credit to Arthur Spirling for the meme idea)
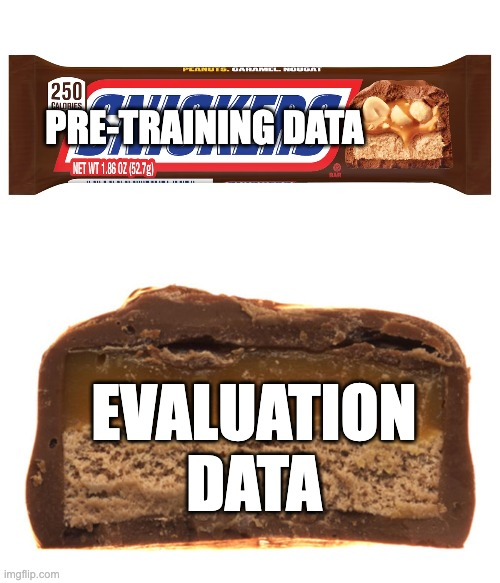
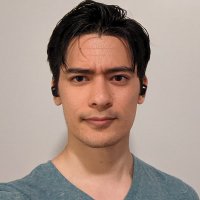
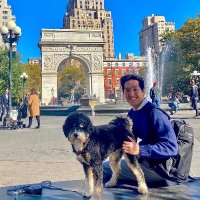
High-quality data is a key to successful pretrain/finetuning in the GPT era, but manual data curation is expensive💸 We tackle data quality challenges involving large models and datasets with ScAlable Meta leArning (SAMA) #NeurIPS2023 💫
Arxiv: arxiv.org/abs/2310.05674
🧵 (1/n)
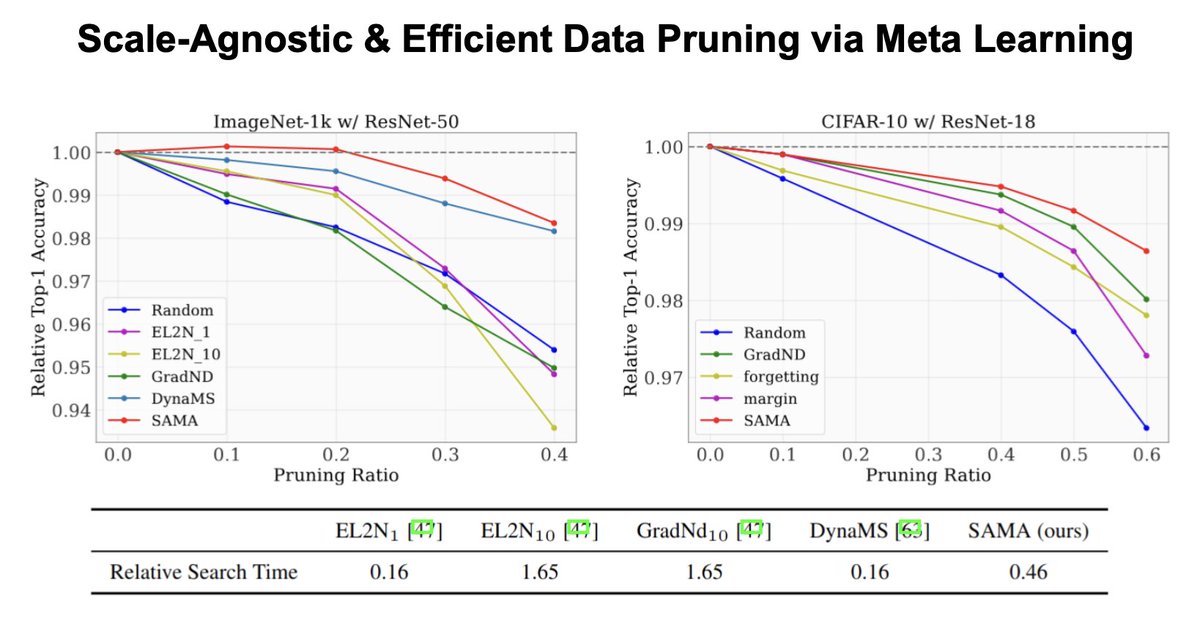

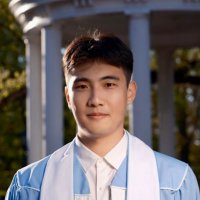
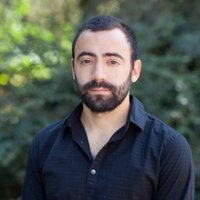
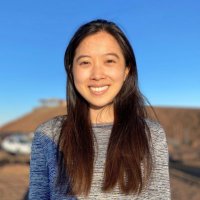
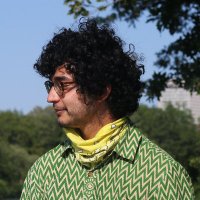
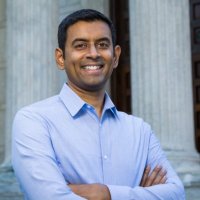


Why does RLHF make outputs longer?
arxiv.org/pdf/2310.03716… w/ Tanya Goyal Jiacheng Xu Greg Durrett
On 3 “helpfulness” settings
- Reward models correlate strongly with length
- RLHF makes outputs longer
- *only* optimizing for length reproduces most RLHF gains
🧵 below:
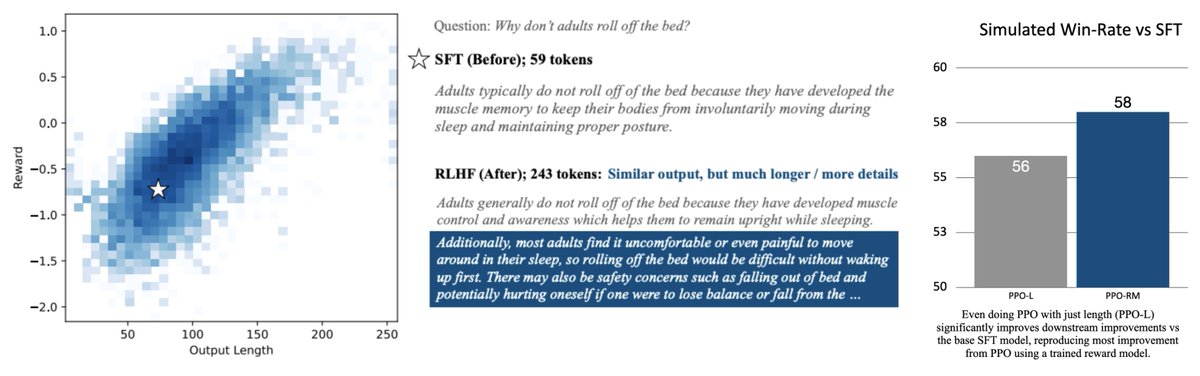
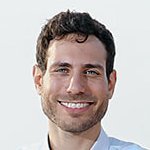
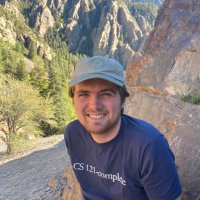
Nucleus and top-k sampling are ubiquitous, but why do they work?
John Hewitt, Alexander Koller, Swabha Swayamdipta, Ashish Sabharwal and I explain the theory and give a new method to address model errors at their source (the softmax bottleneck)!
📄 arxiv.org/abs/2310.01693
🧑💻 github.com/mattf1n/basis-…
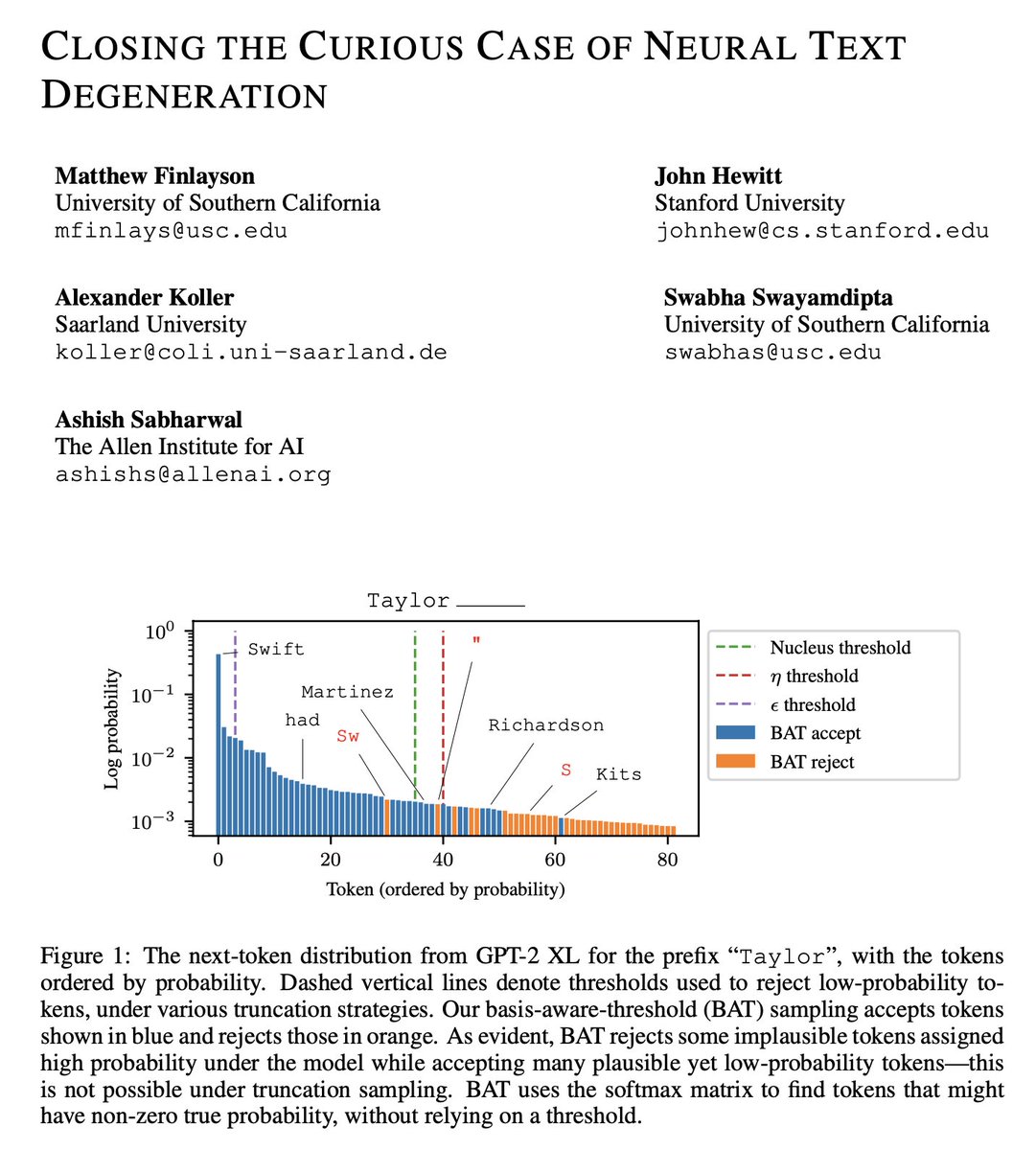